Day 2: Classify Handwritten Digits Using a Simple NN on MNIST
The MNIST dataset is one of the most popular datasets for learning the basics of machine learning and neural networks. It contains handwritten digits (0-9), and our goal is to build a neural network that can classify these digits.
We’ll use a Simple Neural Network (i.e., a Feedforward Neural Network) for this task. Let’s break down the solution into easy-to-follow steps, and I’ll explain every part so you understand what’s happening. We will use Keras, a high-level API in TensorFlow, to make this as simple as possible.
Step-by-Step Solution Outline:
- Load the MNIST Dataset.
- Prepare and Preprocess the Data.
- Build a Simple Feedforward Neural Network.
- Compile and Train the Model.
- Evaluate the Model Performance.
- Make Predictions (optional step to visualize some predictions).
Here’s the full code along with detailed explanations:
Step-by-Step Implementation
Step 1: Import Libraries and Load Data
import numpy as np
import tensorflow as tf
from tensorflow.keras.datasets import mnist
from tensorflow.keras.models import Sequential
from tensorflow.keras.layers import Dense, Flatten
import matplotlib.pyplot as plt
- numpy: Used for numerical operations.
- tensorflow: We’ll use Keras, which is a part of TensorFlow, to create our neural network.
- mnist: The MNIST dataset is built into Keras, which makes it easy to load.
- Sequential and Dense: These help in building a neural network. Sequential is used for stacking layers.
- matplotlib: Used for visualizing the digits from the dataset.
Step 2: Load the MNIST Dataset
# Load the MNIST dataset
(X_train, y_train), (X_test, y_test) = mnist.load_data()
# Check the shape of the data
print(f"Training data shape: {X_train.shape}, Training labels shape: {y_train.shape}")
print(f"Testing data shape: {X_test.shape}, Testing labels shape: {y_test.shape}")
- mnist.load_data() loads the MNIST data and splits it into training and testing sets.
- X_train: Images of handwritten digits used for training the model.
- y_train: Corresponding labels for the training images (digits 0-9).
- X_test and y_test are the images and labels used for testing.
- Shapes:
- X_train.shape: The shape is (60000, 28, 28), which means we have 60,000 images, each with a size of 28x28 pixels.
- y_train.shape: We have 60,000 labels corresponding to the training images.
Step 3: Preprocess the Data
# Normalize the data to range 0-1
X_train = X_train / 255.0
X_test = X_test / 255.0
# Flatten the images from 28x28 to 784 (since a dense layer expects a vector input)
X_train = X_train.reshape(-1, 28 * 28)
X_test = X_test.reshape(-1, 28 * 28)
- Normalize the Data:
- X_train / 255.0: The original pixel values are between 0 and 255. Dividing by 255 scales these values to between 0 and 1, which helps the neural network learn faster.
- Flatten the Images:
- The MNIST images are 28x28 pixels, which we need to flatten into a vector of 784 pixels (28 * 28). This is because a fully connected (Dense) layer expects 1D vectors rather than 2D images.
Step 4: Build the Neural Network
# Build a simple feedforward neural network model
model = Sequential()
model.add(Dense(128, activation='relu', input_shape=(784,))) # First hidden layer with 128 neurons
model.add(Dense(64, activation='relu')) # Second hidden layer with 64 neurons
model.add(Dense(10, activation='softmax')) # Output layer with 10 neurons (for digits 0-9)
- Sequential(): We create a Sequential model to stack layers one after another.
- Dense(128, activation=‘relu’, input_shape=(784,)):
128 neurons in the first hidden layer with ReLU activation function.
- input_shape=(784,) tells the model that the input will be a vector of length 784 (flattened image).
- Dense(64, activation=‘relu’): Adds a second hidden layer with 64 neurons and ReLU activation.
- Dense(10, activation=‘softmax’): The output layer has 10 neurons, each representing one of the digits 0-9.
softmax
activation is used to turn the output into probabilities, with the sum equal to 1.
Step 5: Compile the Model
model.compile(optimizer='adam', loss='sparse_categorical_crossentropy', metrics=['accuracy'])
- optimizer=‘adam’: The Adam optimizer is a popular choice for training deep learning models.
- loss=‘sparse_categorical_crossentropy’: We use categorical cross-entropy since we have multiple classes (0-9) to predict. Sparse is used since our labels are integers (0-9) rather than one-hot encoded vectors.
- metrics=[‘accuracy’]: We use accuracy to track the model’s performance during training and testing.
Step 6: Train the Model
history = model.fit(X_train, y_train, validation_data=(X_test, y_test), epochs=10, batch_size=32, verbose=1)
- model.fit(): Train the model using the training data.
- validation_data=(X_test, y_test): Evaluate performance on the testing data during training.
- epochs=10: Train for 10 complete passes through the training dataset.
- batch_size=32: Update weights after every 32 samples.
- verbose=1: Print detailed information during training.
Step 7: Evaluate the Model
test_loss, test_accuracy = model.evaluate(X_test, y_test)
print(f"Test Accuracy: {test_accuracy:.2f}")
- model.evaluate(X_test, y_test): Evaluates the model’s performance on the test data.
- test_accuracy: This gives us an idea of how well the model can classify unseen handwritten digits.
Step 8: Visualize Training and Validation Performance (Optional)
# Convert history to DataFrame and plot accuracy and loss
history_df = pd.DataFrame(history.history)
history_df[['accuracy', 'val_accuracy']].plot()
plt.xlabel('Epochs')
plt.ylabel('Accuracy')
plt.title('Training and Validation Accuracy')
plt.show()
- pd.DataFrame(history.history): Converts the training history to a DataFrame for easy visualization.
- Plotting:
- Training and validation accuracy are plotted to see if the model’s performance improves over epochs and whether it overfits or underfits.
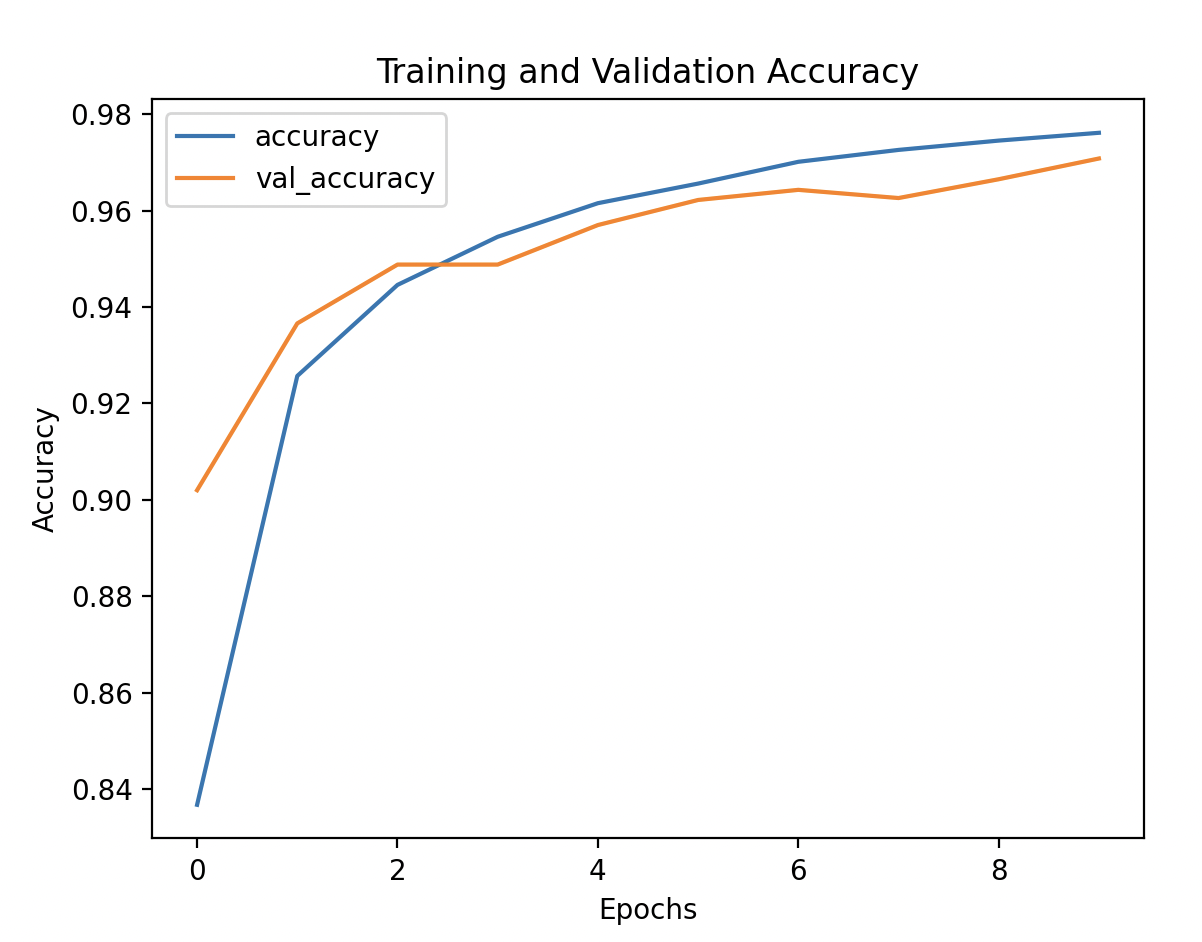
Step 9 (Optional): Make Some Predictions
# Make predictions on the first 5 test images
predictions = model.predict(X_test[:5])
# Display the first 5 images with predicted and true labels
for i in range(5):
plt.imshow(X_test[i].reshape(28, 28), cmap='gray')
plt.title(f"Predicted: {np.argmax(predictions[i])}, True: {y_test.iloc[i]}")
plt.axis('off')
plt.show()
- model.predict(X_test[:5]): Predicts the labels for the first 5 test images.
- plt.imshow(): Displays each of the test images.
- np.argmax(predictions[i]): Retrieves the predicted label for each image.
- y_test.iloc[i]: Shows the true label.
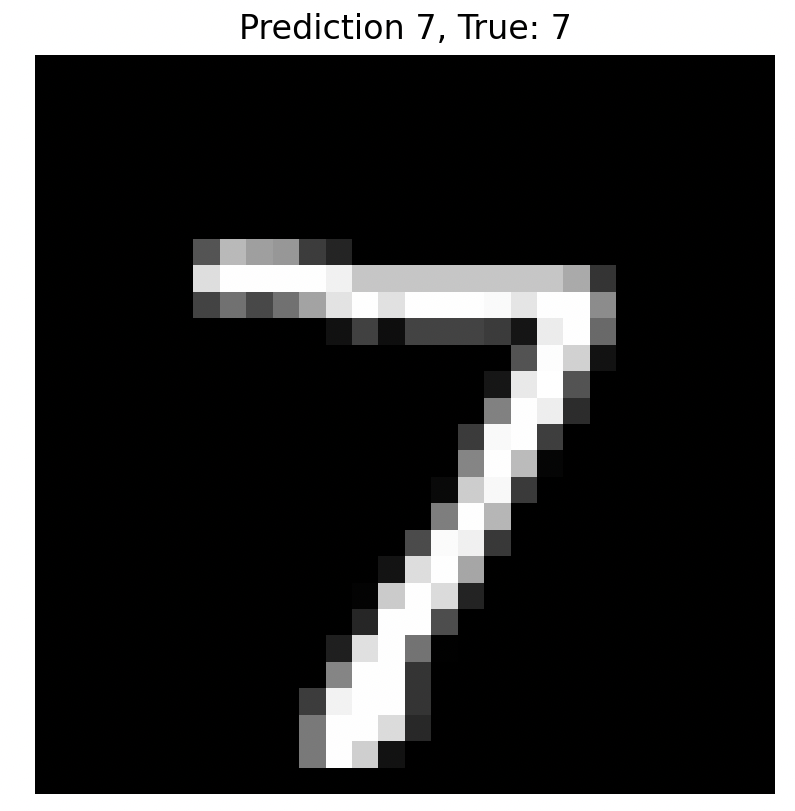
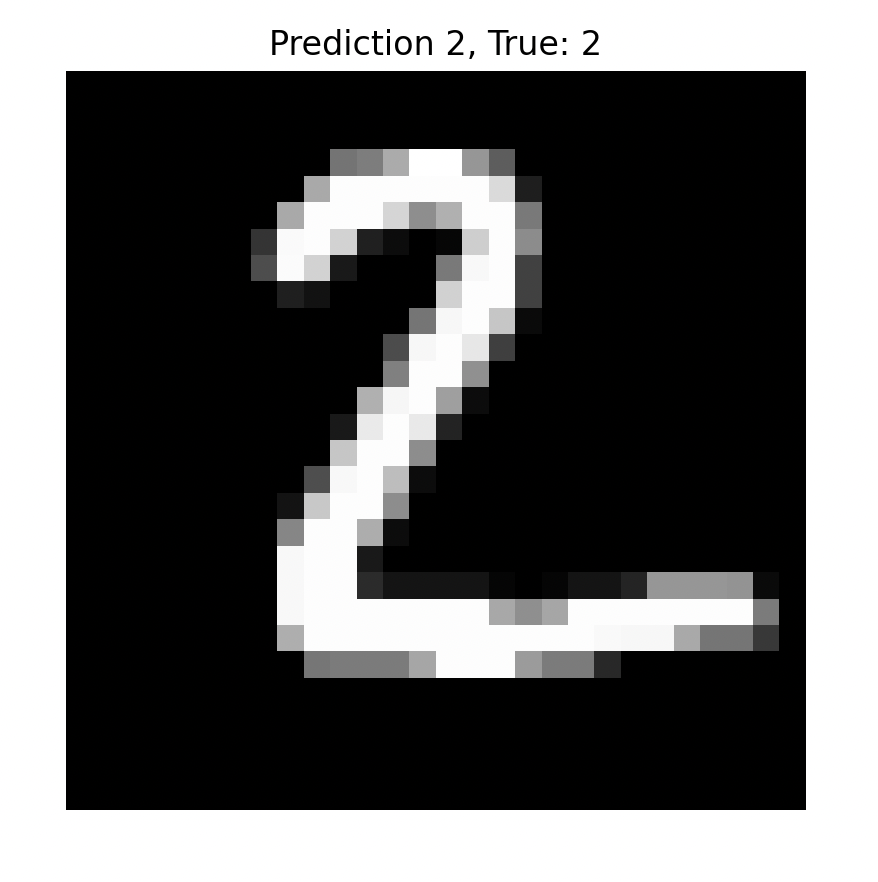
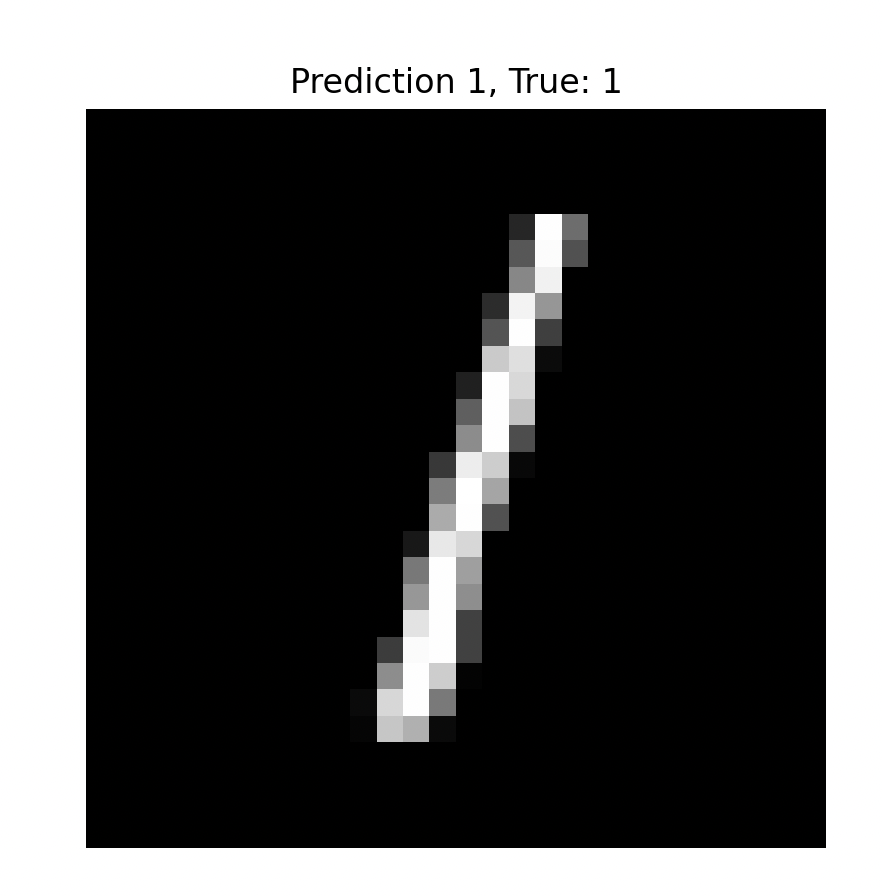
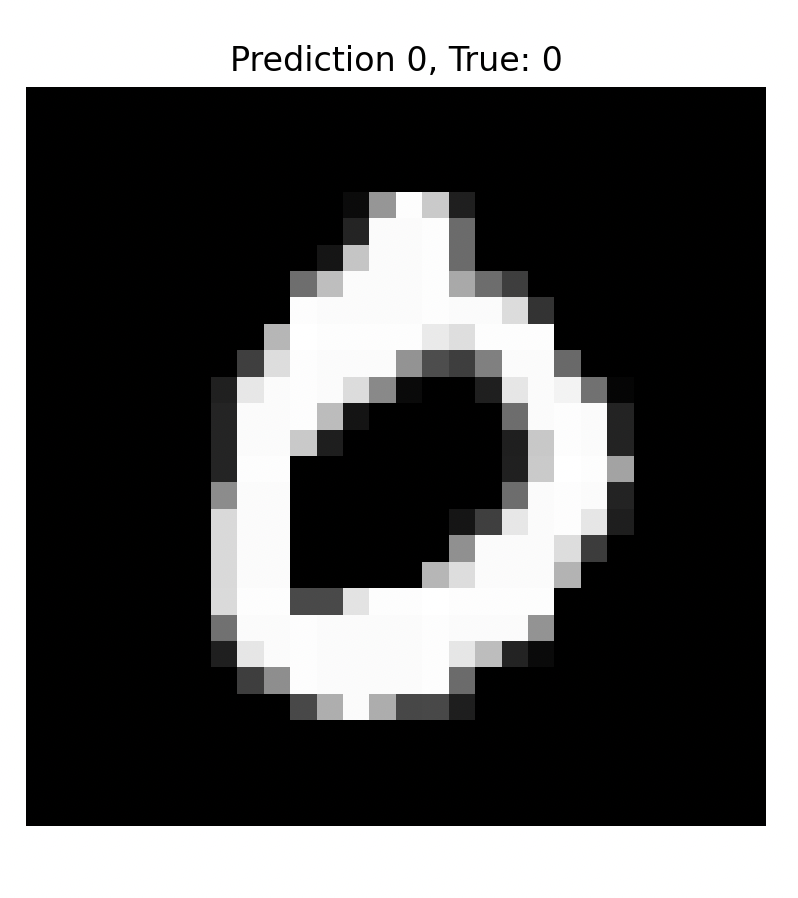
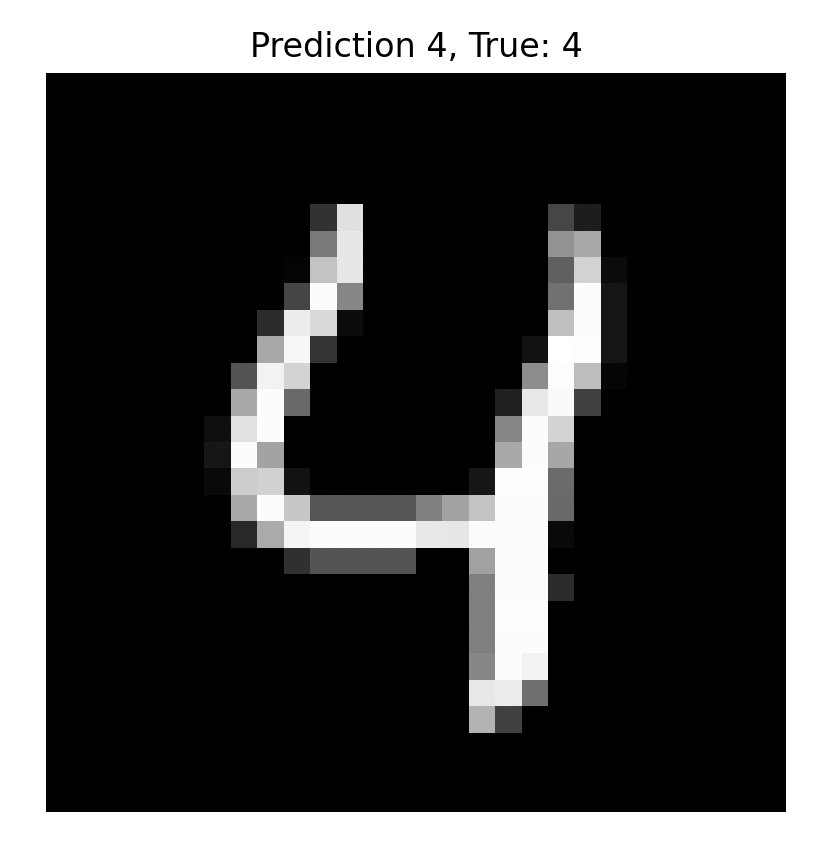
Video
Coming Soon.